Reproducibility checks are of great value for research quality. But which aspects should be given particular attention? Prof. Arthur Seibold reports on his experiences with reproducibility checks and names two aspects on which he places a particular focus.
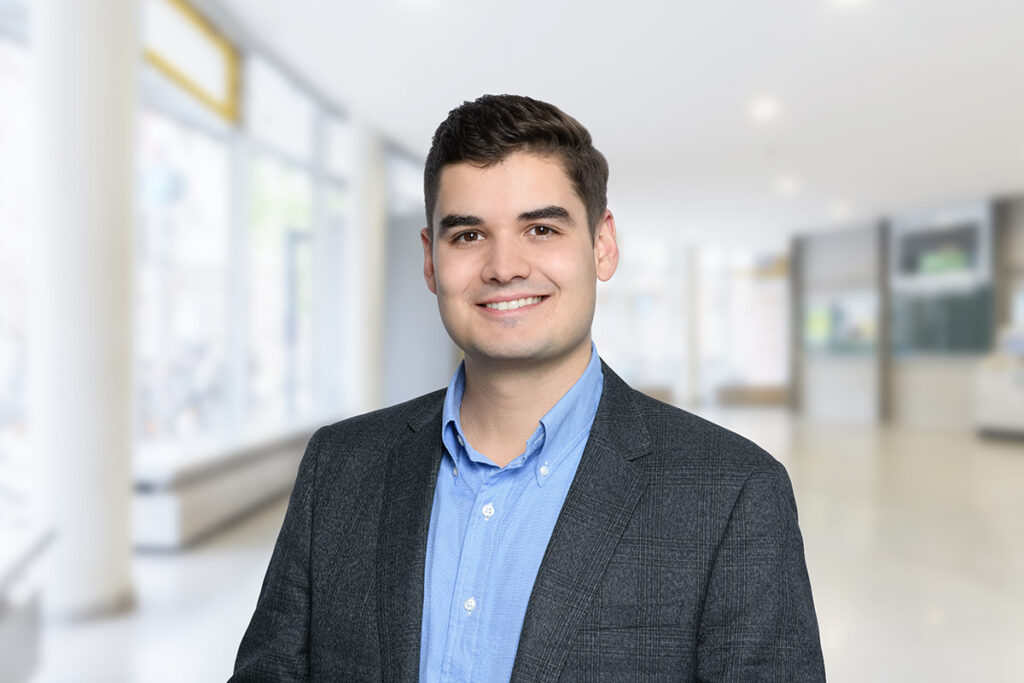
Replicable or reproducible research results are a central aspect of good scientific practice. Non-reproducible or replicable research results are of less benefit and can reduce trust in science (replication crisis).
The reasons for making your own research reproducible and how research is made reproducible are described in the Open Economics Guide.
Prof. Arthur Seibold, Ph.D., Junior Professor of Finance at the University of Mannheim, gives tips and an insight into his experiences with reproducibility checks in this interview.
What do you pay attention to in order to ensure the reproducibility of your research results? Which aspects are particularly important for your research practice?
A large part of my own research is empirical. I work with very extensive administrative data sets, such as German social security data. Reproducibility is often a challenge due to the complexity of these projects and data protection requirements. Basically, I pay attention to two aspects that are also the focus of the replication rules of the most important journals in economics.
The first aspect is data access. Ideally, the research data used should be made publicly available at the latest at the time of publication. Sometimes, however, this is not possible due to data protection regulations – especially when working with sensitive administrative data. In this case, all necessary information should be provided to enable other researchers to access the original datasets. This includes, for example, precise dataset descriptions, specific steps for data access and contact persons on the part of the data providers.
The second aspect is the provision of the codes used by the statistical software (for instance Stata or R) to implement the empirical analysis. On the one hand, these programmes generate the analysis data from the original data and, on the other hand, these programmes generate the empirical results (tables, graphs, etc.). The provision of these codes enables exact reproducibility of the empirical analysis.
What experiences have you had with reproducibility checks?
I have had positive experiences with the procedures of economics journals for the reproducibility of empirical analyses in recent years. However, such reproducibility checks can lead to a considerable amount of work and time spent, both for journals and for authors.
A concrete case to illustrate this: In 2021, I published a paper in the American Economic Review that was subject to the Data and Code Availability Policy of the American Economic Association (AEA). Authors must therefore submit their replication package (data and codes) to the AEA data editor before publication. The replication package is then thoroughly checked by the data editor's team as part of a "reproducibility check". This usually involves running the submitted codes on the submitted datasets to check that the empirical results in the paper can be reproduced. As my paper was based on administrative social security data, I was unable to submit the data and so a full reproducibility check was not possible. Instead, all programmes were checked "manually", i.e. they checked whether the code structure matched the data and results in the paper. Due to the effort involved and some revisions necessary on my part, this process took a total of 4 months, after the paper had previously been in the "revise & resubmit" process with the scientific editor for approximately 12 months.
In the end, these reproducibility checks are of great benefit to research quality, as the complete reproducibility of empirical results is ensured. In addition, replication packages are a valuable resource for researchers who want to use certain data or empirical methods. For good reason, more and more journals are introducing such data and replication rules. However, one should not forget that these involve a great deal of effort for journals and authors.
What tips would you give other researchers?
For me personally, the most important tip is to think about future reproducibility from the outset when working on empirical research projects. When managing data, for example, you should make sure to document all the original data and access modalities and to keep different versions of the data. You will have to make this information available later anyway. For empirical analyses, it is important that intermediate results are documented and reproducible at all times. I also find a certain degree of structure and organisation of the codes helpful. For complex projects with several co-authors, platforms with a version control function, such as GitHub, may be useful.
We would like to thank Prof. Arthur Seibold, Ph.D. for the interview.
Prof. Arthur Seibold, Ph.D., is Assistant Professor of Public Economics in the Department of Economics at the University of Mannheim. His research interests lie in the fields of public finance, labour economics and behavioural economics. He received his Ph.D. from the London School of Economics (LSE).